It's quickly becoming apparent that Integrating AI in your VC operations is no longer the question. AI is here to stay, and just as it has revolutionized most industries, it will also do so in venture. Correction, It’s already doing so. And those who don’t embrace this change risk their relevancy.
The question in many heads
The question, then, is: To remain relevant, should I build or should I buy?.
Is building our own tools and AI solutions to improve your operations the right path? Is it even a possibility at all worth considering?. At Katapult we asked ourselves this question 4 years ago and initiated a path of development that exceeded our (quite humble) expectations back then. With a simple mandate of “staying relevant on AI”, at the time we didn't know the tools and solutions that would lead us to build a platform to support all VC operations from scouting to exit.
Here, I gather some of our learnings and the arguments behind why I believe building is not suitable for most investors. Maybe with the exception of investors with multi billion funds and management fees.
Unsurprisingly, competence is the limiting factor
It’s easy to undervalue what would be needed to build your own AI tools. After all, this tech seems to be everywhere. To be successful you need to have the right people, mindset, approach, and culture, but without the resources you won’t get far. The bottom line is that going down that path is resource intensive, you need to pay for Team of data scientists, Data it self, the Infrastructure, and the Time.
- You need a good technical team who is also savvy in the ways of VC.
- You need to afford the infrastructure costs.
- You need to pay for data (because you need far more data than what you have).
- You need to allow for a learning curve and failed experiments.
- You need an internal culture that welcomes new ways of work.
- And to understand that the budget will hardly have any ROI in the short & mid term.
The capacity to build own tools increases with fund size (as a proxy for management fees, & cash flow).
The smaller the fund size the bigger the benefit.
The paradox lies in that while building their own tools is hardly the achievable choice for most early stage investors. Early stage is where the benefits of AI are more ripe to be substantial, and for now, where you find the biggest gains.
The number of startups at pre-seed and seed is substantially larger than at any subsequent stage. And the number of investors in early stages outnumber the later stages as well.
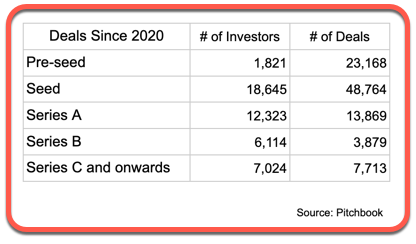
Based on deals registered by Pitchbook alone, the numbers are to big for manual crunching. Between 2020 and today, the count of pre-seed and seed stage deals was close to 70 thousand, while Series A accounted for ca. 14 thousand, and Series B close to 4 thousand. Early stage deals represent up to 10 times the number of deals in later stages, and the number of investors investing in early stage, -not counting angels- is somewhere between 4 to 6 times larger in pre & seed stage than any later stages. Throw in the Angel army, and the ratio is even bigger.
Over the last 10 years, close to 180 thousand deals registered have been closed at pre & seed stages. Using a simple 1% conversion rate from screen to portfolio, one could guesstimate that more than 18 million companies have been screened for those deals to come through, at an average of 4 investors per deal, the number of analyzed deals done by the investing community is monumental.
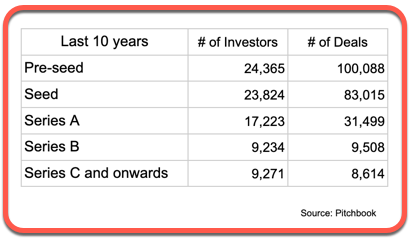
If millions of companies & deals are created every year, being thorough in evaluating all possible deals would require an army of humans that would simply blow out any budget of an early stage investor. This is one of many reasons for why AI disrupts the space: AI scouts and AI analysts can see ALL the deals, in a fraction of the time, and come out with only relevant deals for a human to review. Algorithms don’t get tired like humans do, and are, …well… “a little bit” faster, their learning curve is faster, and their expertise is not lost as it would with the next wave of scouting interns.
At later stages the count of available deals is less, but the case for AI is different, more data is available, and “winner-picking” algorithms are “easier” to develop.
So, if the benefits are so ripe, particularly in the early stage investing, why is it not a good idea for most investors to build their own algorithms?.
Time, Cost, and Timing.
3 reasons explain why building tools may not be the way to go for most. Time, Cost, and Timing.
Time
The VC learning curve is long, talent that is technically capable will hardly have experience in the VC industry, and will need some time to get acquainted and understand the value they can provide.
However, this is assuming the engineers are able to get mentoring, participate in IC meetings, listen to calls and investment decisions, and get good comprehensive feedback from engaged users of their tools and ideas. In reality, more often than not, this knowledge is unintentionally gated, or not seen as a strategic / required activity by the investment talent.
Additionally, GP’s patience can play against. Even though VC is a long waiting game, investors can be impatient when it comes to internal operations. We want things done quickly, and right, and to quickly see the return of our efforts and projects.
Finally the time to develop tools, build the fundamentals, develop the data warehouses, acquire reliable data sources, engineer data pipelines, build algorithms, develop user interfaces, obtain feedback, and iterate is long. Way too long for most.
Cost
Building these tools is not cheap. You’ll need to pay for Talent, Data, and Infrastructure.
Talent: you need specialized AI engineering talent which happens to be the hottest human resource in the world. - Not cheap. You need leaders to start these projects, translate business argot into tech argot and vice versa, manage a team that does nothing similar to the operations of a VC -hard to find-. This is specialized talent you don’t have, and hardly know how to hire.
Data: No matter how big is your excel, or how many contacts you have in your CRM, you simply do not have enough data. Getting access to high quality data, in the reliable form your team will require is not cheap. To be able to collect and scrape what you need, we are talking about hundreds of thousands of euros.
Infrastructure: Building and maintaining your algorithms requires infrastructure and fixed costs that can quickly get out of control. Depending on the volume of data & computational capabilities you require this can easily become a large chunk of your recurring costs.
While your costs will quickly increase, your capabilities will lag, and it will take 2-4 years for the first successful and tangible tools that provide true competitive advantage.
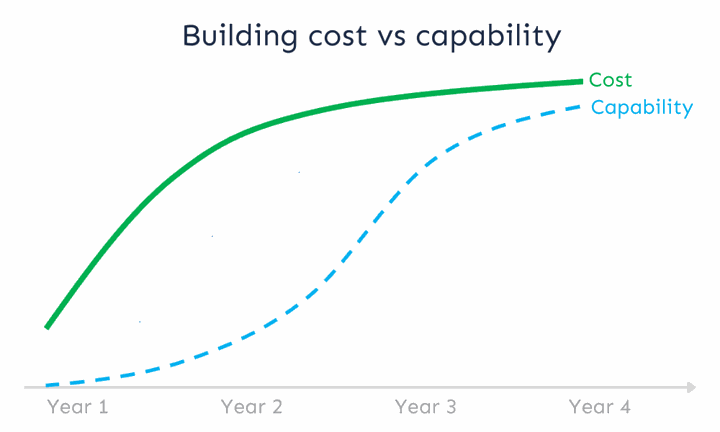
Timing.
Finally, other funds and startups such as Katapult & NorthStar, and EQT with Motherbrain, Red River West with RAMP or Early Bird with Eagle have been working on tools like these for 4+ years, and some are now in place to provide these tools to other investors. For new funds, developing from scratch simply may be too late to get to the starting line.
The advantage of volume
There are other practical challenges to building your own tools. The narrowness of scope and volume of a single fund or few investors means it's harder to iterate or receive diverse feedback from users. Diverse feedback is critical to develop the tools at a pace that allows it to keep on par with other tools or build the right features to remain competitive.
One of the main advantages of having a tool that is co-created with input from many different investors, is that the larger feedback volume and increased diversity of ideas, needs, contexts, and inputs, allows the developer team to build better and iterate faster. New features are more powerful, and the overall value is significantly boosted by the power of many.
From a pure data science perspective, having much more volume of analysis/transactions and a larger use of algorithms, allows engineers to iterate and improve the algorithms and learn new things that are out of the reach with lower volume of transactions.
One final element to consider is that the lack of technical expertise in senior management could lead to developing tools that may not be up to their full potential, or on the opposite side, management could have unrealistic expectations of the tools, leading to an unfair evaluation, disappointment, or prematurely ending the projects before tangible outcomes are real. Wasting valuable resources.
Pooling efforts
Since early stage investors rarely have the size/fees to afford a 2 to 4 million bill to spend in the incremental OPEX that a true internal data-driven initiative would bring to their fund over the next 3-5 years. Building an AI / data driven tool can only be done by pooling resources and partners, with the challenges that this ensues. Or using existing solutions. Which leads to “buy” being the most sensible call.
But how to select what to buy?
We are obviously biased here. But from multiple conversations with different investors trying to build their data-driven tech stack I’ve come to find these key questions one needs to ask when acquiring tools.
- Is this tool for venture investors like me? Do they understand venture?
- Do they truly understand the operational pain points, and is it solving a broad array of issues?
- Is there a sensible future development plan for these tools? Or am I locking in to a tool that will not evolve towards my future needs.
- And for impact investors. Does this tool help me understand and achieve my impact mandate?.
A platform for impact investors, from impact investors.
NorthStar Impact Analytics aims to provide a comprehensive suite of data driven tools for impact investors. Born from within venture to provide solutions to key pain points of daily operations in early stage investing, NorthStar has been developed with a wide array of input from numerous experienced and successful funds and leading European impact and climate investors.
NorthStar’s tools focus on solving the key processes of investment operation: Source, Screen, Select (DD), Support and Sell (The Northstar 5Ses), as well as the Key S enabler (Secure LPs to your fund).
With tailored tools for impact investors that generate state-of-the-art impact assessments used now by renowned impact consultants, and a unique capacity to recommend highly relevant deals to any impact orientation, NorthStar IA is positioned to provide the perfect support for investors who wants to stay at the right side of the future.
No matter where you are in your VC life-cycle. Whether you are in the process of raising your fund, sourcing deals, or supporting your founders and building value in your portfolio, when you buy into NorthStar you will solve your immediate need. Created by a team that from first hand knows what early stage investing is about.
Stay in the loop
To learn more about our tools and suite of solutions, or simply to stay up-to-date sign up for our newsletter here. And if you are ready to become an investor that leverages on the power of AI to augment your operations, try our suite of AI tools to for impact investors. Click here!